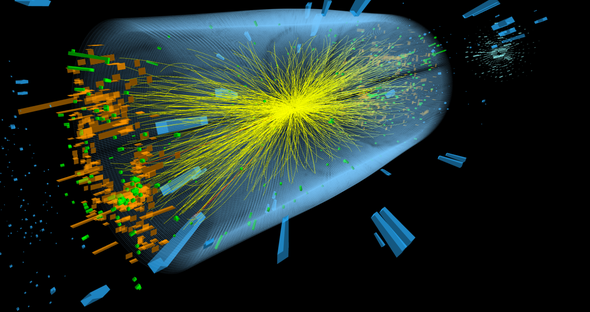
MIT
By: Sang Eon Park
Particle physics poses some of the most fundamental questions humans have about nature. What are the foundational particles that make up the universe? What are the laws that govern the interactions between those particles?
The Large Hadron Collider (LHC) at CERN (the Conseil Européan Pour la Recherche Nucléaire), was built to answer these questions.
The LHC forces particles to collide with one another at a speed of 99.9999991 percent the speed of light. The collisions result in explosions that enable researchers to discover and study new particles fundamental to man’s understanding of matter. The Higgs Boson is one such particle and the confirmation of its existence in 2012 was heralded as a major scientific breakthrough because its function in matter is to give mass to other fundamental particles like electrons.
By forcing these collisions, the LHC generates up to a petabyte (PB) of data per second.
The data represent the trajectories of each accelerated and collided particle. Today, the LHC has generated more than 400 PB of data. Due to this vast data size and the rarity with which interesting events like the Higgs Boson occur, physicists have devised various selection algorithms to help them identify even remotely interesting events to save for further study. With recent advances in machine learning (ML) technology, physicists have refined their selection algorithms to more accurately identify interesting events hidden within these enormous datasets. ML has also enabled other searches that were previously impossible like identifying hadronic decays of the Higgs Boson.
As members of MIT's high energy physics group and the National Science Foundation’s (NSF) Institute for Artificial Intelligence and Fundamental Interactions (IAIFI), our research focuses on the intersection of artificial intelligence (AI) and collider physics data analysis. We develop ML algorithms and methods that can be directly applied to analyzing data from particle colliders.
In 2022, we developed a novel approach to analyze data derived from the LHC. We became the first team to use neural embedding in the field of high energy physics. Specifically, our approach includes the hyperbolic embedding of tree-like structures. We hypothesized that since these particle jets are similar to hierarchical graphs, embedding into a hyperbolic space would work the best. We developed a small transformer model with attention mechanism complete with around 2 million parameters. For reference, the widely known and topical GPT4 has around one trillion parameters.
To deploy this model and analyze a large set of data, we chose Crusoe Cloud because of its simple user interface, reliability, and positive environmental impact. The primary factor driving our selection of Crusoe Cloud is its ease of use. Crusoe makes it extremely easy to set up an account, spin-up a virtual machine (VM), and start training ML models right away. With Crusoe Cloud, we were able to select and spin-up an 8 x NVIDIA A40 48GB node in under three minutes. The user interface is designed with the user squarely in mind and the certificate setup is simple and efficient. In total, it took less than five minutes to start training models.
Crusoe also offers us a robust and reliable cloud platform to conduct our research. We completed days-long training runs on Crusoe Cloud across multiple GPUs with no challenge. We trained around 100 models and ran experiments on 6 benchmark datasets continuously for 10 days with one 8 x A40 GPU node and one 4 x A100 40GB GPU node. We chose to run many models on many A40 and A100 GPUs because the transformer models we used are very sensitive to different initializations. We therefore wanted to run many experiments efficiently to find the setup that gave us the best empirical results for particle physics embedding tasks. The training ran smoothly without any hitches and we encountered no resets to the machines, and only one unrelated maintenance event.
Finally, Crusoe’s positive environmental impact is another attraction. Training big machine learning models takes many GPU hours and is energy intensive. Globally, data centers currently require around 500 million Megawatt hours (MWh) of power annually. Unlike other cloud providers which rely on the electrical grid, Crusoe uses natural gas that would otherwise be wastefully burned off in flares with no beneficial use to power its infrastructure. Crusoe’s patented Digital Flare Mitigation (DFM) technology, takes wasted gas that is composed mainly of methane from oil fields and converts it into electricity. Compared to flaring, which on average has a combustion efficiency of only around 91%, DFM has a combustion efficiency of 99.9%. As methane is more than 80x more potent than CO2, reducing methane emission by 9% via DFM results in up to 69% less CO2e. It’s reassuring to know that by using Crusoe Cloud, we can conduct our research, driving future innovation, without negatively affecting the environment.
We plan to use Crusoe Cloud in the future to conduct more research and data analysis.